Om data verkligen är den nya oljan är vi fortfarande långt ifrån att behärska vetenskapen att utvinna, raffinera och distribuera den som en strategisk företagstillgång. Detta verkar kort sagt vara den slutsats som dras av två olika undersökningar. Detta kan bero på de analytiska utmaningar som följer med IoT-data.
Den första studien från Gartner klassificerar 87% av företagen som företag med låg BI- och analysmognad. Organisationer inom denna låga mognadsgrupp delas vidare in i två nivåer: en grundläggande nivå som kännetecknas av kalkylbladsbaserad analys och en högre opportunistisk nivå där dataanalys används men fragmentariskt och utan något centralt ledarskap och vägledning.
I den andra studien från New Vantage Partners medgav en majoritet av koncerncheferna att de ännu inte hade skapat vare sig en datakultur eller en datadriven organisation. Mer oroande är att andelen företag som identifierar sig som datadrivna tycks minska
Företagen kanske ännu inte är datadrivna, men dataflödet visar inga tecken på minskning.
Enligt IDC kommer den globala datasfären att växa till 175 Zettabyte (ZB) år 2025, en ökning från 23 ZB år 2017. Även om så sker kommer konsumenternas andel av dessa data att minska från 47% 2017 till 36% 2025. Detta innebär att den största delen av denna dataström kommer att drivas av det som IDC kallar den sensoriserade världen eller IoT.
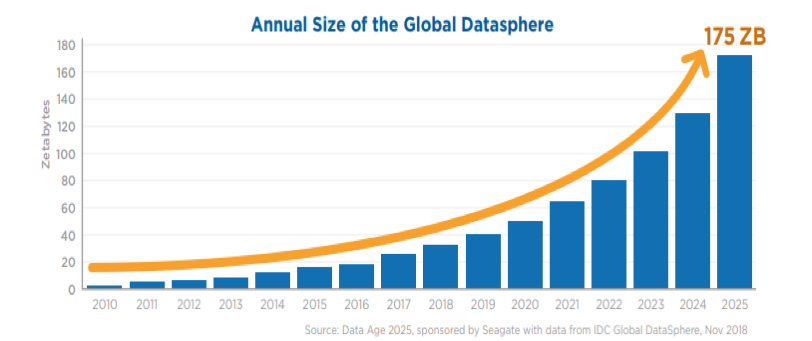
Viktiga utmaningar med IoT-data
Ökningen av IoT-data har ett stort ekonomiskt värde, som uppskattas till cirka 11 biljoner USD år 2025. Men det innebär också stora utmaningar när det gäller att samla in data från olika, distribuerade källor och använda analyser för att utvinna strategiskt värde.
Den främsta utmaningen med IoT-data är dess realtidskaraktär. År 2025 kommer 30% av all data att vara realtidsdata, varav IoT står för nästan 95%, 20% av all data kommer att vara kritisk och 10% av all data kommer att vara hyperkritisk. Analys måste ske i realtid för att företagen ska kunna dra nytta av dessa typer av data.
Sedan har vi frågan om tidsseriedata. Detta avser alla data som har en tidsstämpel, t.ex. en smart mätningstjänst inom IoT-området eller till och med aktiekurser. Ett företags IoT-infrastruktur måste kunna samla in, lagra och analysera enorma mängder tidsseriedata. Utmaningen här är att de flesta konventionella databaser inte är utrustade för att hantera denna typ av data.
Den distribuerade karaktären hos IoT-data, där de flesta data skapas utanför företagens datacenter, innebär också en egen uppsättning analysutmaningar. Det främsta är behovet av att bearbeta åtminstone en del av dessa distribuerade data, särskilt hyperkritiska och kritiska data, så att de kan bearbetas utanför datacentret. Själva IoT-analysen måste därför bli distribuerad och en del av analyslogiken flyttas från molnet till edge. IoT-analyser kommer att behöva distribueras över enheter, edge-servrar, gateways och centrala bearbetningsmiljöer. Faktum är att Gartner förutspår att hälften av alla stora företag kommer att integrera edge computing-principer i sina IoT-projekt senast 2020.
Detta är bara några av de egenskaper hos IoT-data som skiljer dem från konventionella datamängder. Och traditionella tekniker och funktioner för dataanalys är inte utformade för att hantera volymen, variationen och komplexiteten hos IoT-data. De flesta företag måste helt förnya sin analyskapacitet för att inkludera IoT-specifika funktioner som streaminganalys, förmågan att identifiera och prioritera mellan olika datatyper och -format samt edge-analys.
CSP:er tar ledningen inom IoT-dataanalys
Många företag vänder sig till molnbaserade IoT-plattformar som erbjuder omfattande datatjänster vid sidan av sina IoT-kunsterbjudanden. Kunderna efterfrågar realtidsfunktioner för datainsamling, lagring, bearbetning och analys, t.ex. för datainsamling, transformation, lagring och bearbetning. Vissa molnleverantörer erbjuder till och med sin egen hårdvara för att förbättra interoperabiliteten och prestandan mellan IoT-enheter och data som bearbetas i molnet.
Enligt en studie från Bain & Company ses CSP:er (molntjänstleverantörer) som ledande när det gäller att tillhandahålla en omfattande uppsättning verktyg som tillgodoser företagets alla behov av IoT-dataanalys. Enligt samma studie spelar dessa CSP:er också en viktig roll för att minska hindren för IoT-användning, underlätta enklare implementeringar och göra det möjligt för kunderna att designa, driftsätta och skala upp nya användningsområden så snabbt som möjligt.
AWS tar ledningen bland CSP:er för IoT
Bland de stora varumärkesleverantörerna har Amazon AWS konsekvent rankats som den plattform som föredras, följt av Microsoft Azure och Google Cloud Platform, i den årliga IoT Developer Survey som genomförs av Eclipse IoT Working Group.
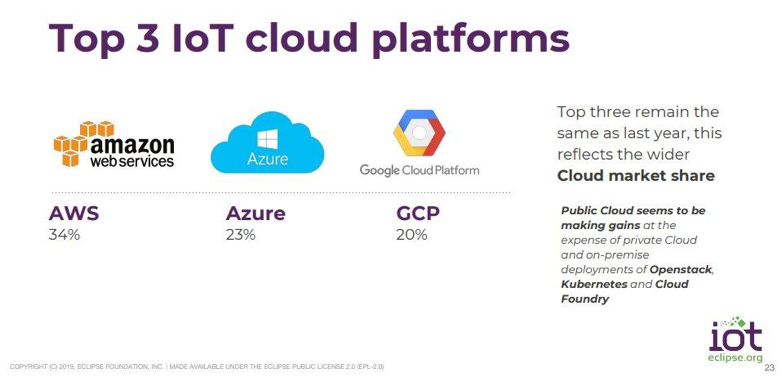
Eftersom datainsamling och analys fortfarande är en av de tre viktigaste frågorna för utvecklare, erbjuder Amazon AWS den mest robusta molnbaserade IoT-analyslösningen på marknaden idag.
AWS IoT Analytics-plattformen är en hanterad tjänst som eliminerar komplexiteten i att driftsätta sofistikerade analyser för massiva volymer av IoT-data. Med AWS Lambda får utvecklare också tillgång till en funktionell programmeringsmodell som gör att de kan bygga och testa IoT-applikationer för både moln och lokala driftsättningar.
När det gäller datainsamling och analys erbjuder Amazon två distinkta tjänster i form av AWS IoT Analytics och Kinesis Data Analytics.
AWS IoT Analytics har de funktioner som krävs för en rad olika IoT-applikationer med inbyggt AWS Core-stöd för att förenkla installationsprocessen. Med AWS IoT Analytics blir det mycket enklare att rensa bort dåliga data och berika dataströmmar med externa källor. AWS IoT Analytics ger datavetare tillgång till rå och bearbetad data, möjlighet att spara och hämta specifika delmängder av data och flexibilitet för regelbaserad dirigering av data över flera processer.
Kinesis Data Analytics är mer lämpat för applikationer för realtidsdatainsamling, såsom fjärrövervakning och processtyrning, som kräver svarstider i millisekundintervallet. Tjänsten integreras med andra AWS-verktyg som Amazon DynamoDB, Amazon Redshift, AWS IoT, Amazon EC2, för att effektivisera dataanalysprocessen. Kinesis Analytics-sviten består av en rad tjänster, inklusive Kinesis Data Streams, Kinesis Data Firehose, Kinesis Data Analytics och Kinesis videoströmmar. Amazon Kinesis Data Streams möjliggör kontinuerlig insamling av stora volymer realtidsdataflöden och händelser av olika slag. Rådata från Kinesis kan sedan rensas och bearbetas via AWS Lambda eller Amazon ECS. Kinesis Firehose förbereder och laddar strömmande data till S3, Redshift eller Elasticsearch för bearbetning och analys i nära realtid.
Medan Kinesis erbjuder utvecklare mer flexibilitet i utveckling och integration, fokuserar AWS IoT på att förenkla distributionen med hjälp av förbyggda komponenter. Det är möjligt att kombinera dessa två lösningar för att bygga en omfattande IoT-lösning som omfattar både strömmande och vilande data.
I slutet av förra året tillkännagav Amazon AWS lanseringen av fyra nya funktioner som skulle göra det enklare att ta in data från edge-enheter. AWS IoT SiteWise är en hanterad tjänst som gör det enkelt att samla in, strukturera och söka data från industriell utrustning i stor skala. Med AWS IoT Events kan kunderna nu enkelt upptäcka och reagera på händelser från ett stort antal IoT-sensorer och applikationer. AWS IoT Things Graph möjliggör en kodfri IoT-utveckling med ett visuellt dra-och-släpp-gränssnitt som gör det möjligt för utvecklare att bygga IoT-applikationer genom att helt enkelt ansluta enheter och tjänster och definiera deras interaktioner. Slutligen hade vi AWS IoT Greengrass Connectors, en tjänst som skulle göra det möjligt för utvecklare att ansluta enheter till tredjepartsapplikationer, lokal programvara och AWS-tjänster via moln-API:er.
Utöver detta har AWS etablerat ett starkt partnernätverk med leverantörer av edge-to-cloud-tjänster och tillverkare av enheter för att erbjuda kunderna den djupaste tekniska och domänexpertis som krävs för att mildra komplexiteten i IoT-projekt.
Förutom att vara en favorit bland utvecklare har AWS IoT också byggt upp en kundlista med några av de största varumärkena i branschen, inklusive LG, Bayer, NASA, British Gas och Analog Devices, för att bara nämna några.
Trots utmaningarna med Big Data och analys har det skett många framgångsrika implementeringar av IoT inom olika sektorer. Här är bara några exempel på hur företag och deras IoT-partners har lyckats utnyttja kraften i analys av stora datamängder inom IoT.
Framgångshistorier inom IoT-dataanalys
Bayer och AWS IoT Core: Bayer Crop Science, en division inom Bayer, tillhandahåller en rad produkter och tjänster som maximerar skördeproduktionen och möjliggör ett hållbart jordbruk för jordbrukare över hela världen. Företaget använder IoT-enheter på skördemaskiner för att övervaka skördeegenskaper som sedan överförs manuellt, under flera dagar, till sina datacenter för analys. Avsaknaden av datainsamling och analys i realtid innebar att Bayer inte omedelbart kunde åtgärda eventuella problem med utrustningskalibrering, störningar eller avvikelser för att hjälpa till med ruttplaner för efterföljande körningar.
Bayers IoT-team, som redan var kund hos AWS, beslutade att flytta sin pipeline för datainsamling och analys till AWS IoT Core. Företaget byggde en ny IoT-pipeline för att hantera insamling, bearbetning och analys av data från fröodling.
Den nya lösningen fångar upp flera terabyte data, i genomsnitt en miljon egenskaper per dag under planterings- eller skördesäsongen, från företagets forskningsfält över hela världen. Denna data levereras till Bayers dataanalytiker i nära realtid. AWS IoT-lösning ger också ett robust ramverk för edge processing och analys som kan skalas över en mängd olika IoT-användningsfall och IoT-initiativ.
Bayer planerar nu att använda AWS IoT Analytics för att samla in och analysera drönarbilder och data från IoT-miljösensorer i växthus för övervakning och optimering av odlingsförhållandena.
Microsoft Azure IoT Hub och ActionPoint: Många tillverkare använder fortfarande papperschecklistor, manuella processer, mänskliga observationer och äldre teknik för slutna kretslopp för att övervaka och underhålla sin utrustning. Även när det gäller moderniserade anläggningar hade tillverkarna ofta inte rätt sensorer på plats för att tillhandahålla all den data som krävdes, eller så hade de inga analyslösningar för att analysera sensordata.
ActionPoint, som utvecklar kundanpassad programvara, samarbetade med Microsoft och Dell Technologies för att utveckla IoT-PREDICT, en industriell IoT-lösning för prediktivt underhåll som innehåller maskininlärning, dataanalys och andra avancerade funktioner. Lösningen drivs av operativsystemet Microsoft Windows 10 IoT Enterprise som körs på Dell Edge Gateway-hårdvara, och kombineras med Microsoft Azure-verktyg för att ge toppmodern edge computing.
Kombinationen av Windows 10 IoT Enterprise och Azure ger en mycket effektiv IoT-lösning som kunderna kan driftsätta på några minuter. Det ger också IoT-PREDICT-lösningen den flexibilitet och skalbarhet som gör det möjligt för tillverkare att börja i liten skala med IoT och växa i sin egen takt.
IoT-PREDICT hjälper tillverkare att snabbt minska stilleståndstiden, sänka kostnaderna och öka den övergripande effektiviteten i sin utrustning och verksamhet. Det hjälper till att maximera effekten av tillverkardata genom att använda Microsoft Azure IoT Hub för att samla in data och göra den tillgänglig för flera Azure-tjänster, inklusive Azure Time Series Insights, Azure Stream Analytics. Tillverkarna kan nu utforska data med hjälp av Time Series Insights eller använda Stream Analytics för att vidta åtgärder med hjälp av data genom att ställa in frågor och varningar baserat på olika prestandatrösklar.
IoT-dataanalys har vissa unika egenskaper och utmaningar som inte kan hanteras med konventionella analystekniker och funktioner. Men precis som i all analysverksamhet är det primära målet detsamma: att generera handlingsbara insikter som kan bidra till ett positivt affärsvärde. Det handlar inte bara om val eller sensor eller anslutningsprotokoll eller CSP. Det måste handla om att säkerställa integriteten i det som McKinsey definierar som värdekedjan för insikter. För att säkerställa att varje IoT-projekt leder till påvisbart affärsvärde måste organisationer säkerställa integriteten i hela värdekedjan för insikter.
Pentair & AWS: Pentair är ett vattenreningsföretag som erbjuder ett omfattande utbud av smarta, hållbara vattenlösningar för hem, företag och industrier runt om i världen. Företaget förlitar sig på uppkopplade system för att övervaka och hantera sina produktinstallationer, varav de flesta finns på avlägsna platser. Traditionellt har företaget använt sig av specialbyggnation för att utveckla sina anslutna system, vilket har medfört en rad nackdelar.
Pentair behövde en kraftfull och flexibel IoT-plattform med hög tillgänglighet och skalbarhet samt en hög grad av återanvändning inom alla verksamhetsområden. Pentair ville också ha en heltäckande lösning som täckte allt från insamling av IoT-data till analys och visualisering.
Företaget samarbetade med AWS Partner Network (APN) Advanced Technology Partner och IoT Competency Partner Bright Wolf för att utvärdera potentiella teknikleverantörer som Amazon, GE, IBM, Microsoft och andra mot en uppsättning plattformsegenskaper. Detta inkluderar datainhämtning, infrastrukturkontroller, driftsättningsalternativ, maskininlärnings- och visualiseringsverktyg, utvecklingsverktyg och den övergripande öppenheten hos varje plattform.
“AWS vann när det gällde den råa poängsättningen”, säger Brian Boothe, ansvarig för Pentairs Connected Products Initiative.
Hittills har Pentair driftsatt tre olika uppkopplade lösningar med hjälp av AWS IoT-plattform och en flexibel, skalbar och återanvändbar referensarkitektur som utvecklats av Bright Wolf. Fördelarna enligt Pentair inkluderar snabbare marknadsintroduktion för mervärdestjänster, enklare integration, kostnadsbesparingar genom att använda vanliga edge-enheter på den öppna AWS IoT-plattformen, skalbarhet och tillgänglighet i företagsklass.